Salivary RNA sequencing highlights a sex-specific developmental time course towards oral feeding maturation in the newborn
Introduction
Attainment of oral feeding competency is a major determinant of length of stay in the Neonatal Intensive Care Unit (NICU) and represents a developmental challenge for the majority of the 15 million infants born prematurely (<37 weeks’ gestational age) worldwide each year (1-3). Inappropriate feeding attempts can lead to acute and long-term morbidities, as well as prolonged hospitalizations with associated healthcare costs (1-6). Furthermore, infants who fail to successfully orally feed by corrected term gestational age (GA) are at increased risk for developmental delays throughout infancy and childhood (4-6). Despite the prevalence of oral feeding morbidities and their long-term health consequences, our ability to assess oral feeding maturity, and more importantly determine the biological mechanisms limiting oral feeding success, remain a clinical challenge (7-10).
Successful oral feeding is dependent upon the simultaneous maturation and integration of the gut-brain axis, as well as sensorimotor, neurodevelopmental and gastrointestinal systems (3,11). Disruption in any one of these systems may vary among newborns, thus a ‘one-size’ fits all approach to treatment strategies and assessment tools to improve oral feeding outcomes is not effective. Cochrane Reviews conducted in both 2012 and 2016 confirmed the futility of available feeding assessment tools for use in the newborn, concluding both times that there is currently “no evidence to inform clinical practice” (8,9).
Variation in the maturation of biological systems involved in oral feeding is believed to be affected, in part, by sex and GA (12,13). Males typically learn to orally feed at older post-menstrual ages (PMAs) compared to females (12), and infants born at earlier GAs learn to orally feed at older PMAs compared to infants born later in gestation (13). In order to provide relevant information to caregivers to personalize treatment strategies and improve oral feeding outcomes, assessment tools must be able to simultaneously evaluate the diverse biological systems required for oral feeding competency and report on the real-time developmental status of an individual newborn.
This study utilized RNA sequencing (RNASeq) in order to advance our understanding of delayed developmental pathways limiting feeding success, identify relevant networks associated with oral feeding competency, and explore sex-specific differences involved in oral feeding maturation in the newborn. Sequencing was performed on whole saliva, a rich source of systemic gene expression (14,15), collected from sex- and age-matched infants who could and could not orally feed. Previously, our laboratory used saliva to discern between successful and unsuccessful neonatal oral feeders utilizing both gene expression microarrays (11), as well as high-throughput, multiplex reverse transcription-quantitative polymerase chain reaction (RT-qPCR) platforms (11,16). Here, we hypothesized that the RNASeq platform would provide a more comprehensive and unbiased analysis of neonatal development, as it relates to oral feeding, and significantly improve our clinical approach to oral feeding difficulties in the newborn. We present the following article in accordance with the STROBE reporting checklist (available at https://pm.amegroups.com/article/view/10.21037/pm-21-45/rc).
Methods
Subject selection and recruitment
This prospective, observational, single-center study was conducted from 2014 to 2017 in the Tufts Medical Center NICU with approval by the Tufts Medical Center Institutional Review Board. The study was conducted in accordance with the Declaration of Helsinki (as revised in 2013). Informed consent was obtained from parents of infants ranging from 34 to 39 weeks’ PMA. There was equal representation of successful and unsuccessful oral feeders. Race of subjects was defined by the parents and recorded in the medical record. The Tufts Medical Center NICU utilizes the cue-based feeding assessment protocol of Ludwig and Waitzman (17). In accordance with this protocol, no infant less than 32 weeks’ PMA is offered oral feeding in the NICU. Infants were considered unsuccessful oral feeders if they took <50% of feeds by mouth (non-feeder); successful oral feeders took 100% of enteral nutrition by mouth and did not have a nasogastric tube in place (feeder) for at least 24 hours. For all demographic data, statistical significance was set at P<0.05.
Saliva collection & quality control
Saliva samples were obtained using previously described techniques (18). Briefly, saliva (~10 to 20 µL) was collected with a 1 mL syringe attached to low wall suction, placed immediately in 500 µL of RNAProtect Saliva (QIAGEN) at the bedside, vortexed, put on ice and stored at 4 ℃ for a minimum of 48 hours up to 4 weeks. Saliva was only collected from a single time point for each subject. Samples were collected during the day and prior to a feed to limit the impact of emerging circadian rhythms on gene expression as well as breast milk contamination. Total RNA was extracted with the RNAProtect Saliva Mini Kit (QIAGEN) per manufacturer’s instructions. On column DNase treatment was performed for each sample to eliminate DNA contamination. Extracted total RNA was stored at −80 ℃ pending quality assessment analysis.
Prior to RNASeq, the quality and quantity of extracted total RNA was assessed on the Agilent Bioanalyzer 2100. Only those samples that met pre-established criteria designed specifically to target cell-free RNA in saliva were subsequently sequenced. Salivary cell-free RNA, as compared to cellular RNA that is largely derived from epithelial and hematopoietic cells within the oral cavity, is believed to be reflective of the systemic body (19), and thus was the target of this analysis. Quality assessment criteria included: (I) a minimum of 500 ng of total RNA per sample; and (II) a RNA Integrity Number (RIN) between 5 and 8, the expected range for cell-free RNA (20).
RNA sequencing
Samples that met qualitative criteria underwent next generation sequencing at the Tufts University Genomic Core facility on the Illumina HiSeq platform. Library preparation was performed with the Illumina TruSeq Stranded Total RNA kit. Ribosomal depletion occurred with the Ribo-Zero Globin kit. Following library preparation, the libraries were denatured, introduced into the flow cell, and subjected to bridge amplification in order to create clonal clusters of single stranded cDNA molecules (21,22). Libraries were sequenced using Rapid V2 chemistry via paired end 150 base format, targeting a sequencing depth of 25 million paired reads or more per sample.
Data analysis
Raw data were obtained in the form of FASTQ files (23). Bioinformatics analyses were performed on the Tufts University High Performance Cluster (Medford, MA). Sequencing quality was assessed with FASTQC (Babraham Bioinformatics, Babraham Institution, Cambridge, UK). Reads were truncated to remove base positions that scored less than a low median score. A median quality score of <20 was deemed unusable. Tuxedo Tools were used to analyze the RNASeq results (24,25). Briefly, reads were mapped to the UCSC hg19 human genome with Tophat 2/Bowtie 2. Normalization and differential expression analyses were performed with Cuffdiff.
Statistical and computational analyses
Data were analyzed in their entirety based upon feeding status, as well as separated by sex. All analyses were conducted using Qlucore with an adjusted false discovery rate (FDR) P value of 0.05. Genes that were differentially expressed between feeding stages were identified and further explored with the use of Ingenuity® Pathway Analysis (IPA) software (QIAGEN Inc, version: 463341M https://www.qiagenbionformatics.com/products/ingunuity-pathway-analysis) (26). IPA utilizes a non-topological based approach to identify over represented genes in a given pathway and was used to identify developmental pathways that were statistically significantly impacted based on feeding status (27). Qlucore was used to perform principle component and hierarchical cluster analyses and 3D Principal Component Analysis (PCAs) and heat map visualizations (28).
Results
Thirty-two infants were recruited for this study; 26 subjects met RNA sequencing quality criteria and ultimately were sequenced (81% success rate). Subject demographics of all subjects who underwent sequencing, separated by feeding status and sex, are summarized in Table 1. Mean RNA sequencing read alignment rates averaged 35% (Table S1). The inclusive analysis of all subjects identified 63 genes that were differentially expressed between the successful (n=13) and unsuccessful oral feeders (n=13). When separated by sex, 88 differentially expressed genes were identified among the female cohorts (n=14), 14 of which overlapped with the original 63 genes identified in the total cohort. Comparatively, 78 differentially expressed genes were identified among the male cohort (n=12), six of which overlapped with the original 63 genes. No overlap of differentially expressed genes was observed between females and males (Figure 1). Differentially expressed genes identified via all three evaluations are presented in Figure 2, while chromosomal location of each gene is provided in Table S2. Ten differentially expressed genes were located on the X chromosome; no genes were located on the Y chromosome. PCAs displayed distinct clustering of successful feeders vs. unsuccessful feeders (Figure 3), with corresponding heat map analyses and gene lists (Figure 4A-4C). The color-coded heat maps depict genes that were up-regulated (yellow) or down-regulated (blue) between unsuccessful and successful oral feeders. Of all three dataset comparisons (total, females, and males), the male subgroup showed the most distinctive clustering based upon feeding status.
Table 1
Demographics based on feeding status | Successful feeders | Unsuccessful feeders | P valuea |
---|---|---|---|
All Infants | |||
Mean gestational age (range), wk | 34.71 (33.1–38.3) | 33.67 (30.4–35.6) | 0.05 |
Mean birth weight (range), g | 2,373 (1,350–3,182) | 2,022 (1,240–2,905) | 0.09 |
Mean post-menstrual age (range), wkb | 35.48 (34.3–39.1) | 35.5 (34.1–39) | 0.93 |
Mean weight at sample collection (range), g | 2,290 (1,410–3,098) | 2,070 (1,555–2,730) | 0.19 |
Race, no. (%) | |||
▪ Caucasian | 92 | 69 | – |
▪ Hispanic | 8 | 15 | – |
▪ Asian | 0 | 8 | – |
▪ Not reported | 0 | 8 | – |
Males | |||
Mean gestational age (range), wk | 34.78 (34.1–36.4) | 33.71 (32.4–35.6) | 0.12 |
Mean birth weight (range), g | 2,496 (2,339–3,079) | 2,122 (1,225–2,905) | 0.27 |
Mean post-menstrual age (range), wk | 34.45 (34.6–36.7) | 35.55 (34.1–37) | 0.88 |
Mean weight at sample collection (range), g | 2,393 (2,049–2,968) | 2,195 (1,617–2,730) | 0.43 |
Ethnicity, no. (%) | |||
▪ Caucasian | 100 | 67 | – |
▪ Hispanic | 0 | 33 | – |
Females | |||
Mean gestational age (range), wk | 34.6 (33.8–38.28) | 33.60 (30.4–35.5) | 0.21 |
Mean birth weight (range), g | 2,250 (1,350–3,182) | 1,924 (1,240–2,279) | 0.20 |
Mean post-menstrual age (range), wk | 35.5 (34.29–39.14) | 35.45 (34.3–39) | 1 |
Mean weight at sample collection (range), g | 2,203 (1,410–3,098) | 1,962 (1,555–2,453) | 0.31 |
Ethnicity, no. (%) | |||
▪ Caucasian | 83 | 72 | – |
▪ Asian | 0 | 14 | – |
▪ Hispanic | 17 | 0 | – |
▪ Not reported | 0 | 14 | – |
a, paired t-test; b, PMA at sample collection.
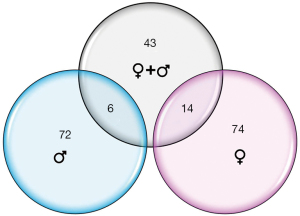
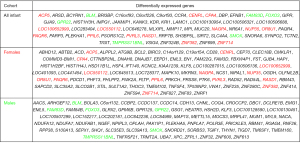
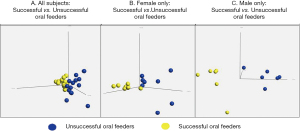
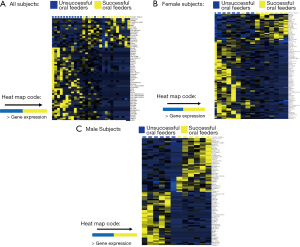
Differentially expressed developmental pathways identified in all three cohort analyses, along with their significant P values and genes, are described in Table 2. The 63 genes that were differentially expressed between all successful and unsuccessful oral feeders, clustered into the following most statistically significant developmental networks: nervous system development and function, tissue morphology, embryonic development, hematologic development and function, and hematopoiesis. Systems biology analyses highlighted genes related to memory and learning, disruption in palatal shelf formation, maturation of circadian rhythms, abnormal morphology of hindgut and mesenchyme and development of the abdomen (29,30). However, when separated by sex, two distinct analyses emerged.
Table 2
Subjects | Developmental pathway | P values and numbers of associated genes within pathway |
---|---|---|
All infants | Nervous system development and function | P values: <0.006 to <0.0001, N=7 genes: EFNB1, FOXO3, LAMC1, NECTIN3, NR6A1, RASD1, SIRT2 |
Tissue morphology | P values: <0.02 to <0.002, N=10 genes: BLM, CCR4, EFNB1, FOXO3, KDR, LAMC1, NECTIN3, NR6A1, SLC4A4, TCTN2 | |
Embryonic development | P values: <0.02 to <0.002, N=12 genes: BLM, EFNB1, FOXO3, GIPR, KDR, LAMC1, MMP17, NECTIN3, NMP17, PLEXHA1, SIRT2, TCTN2 | |
Hematologic development and function | P values: <0.02 to <0.002, N=9 genes: BLM, CCR4, EFNB1, FOXO3, KDR, PLEKHA1, SLC4A4, TIGIT, VSIG4 | |
Hematopoiesis | P values: <0.02 to <0.002, N=6 genes: BLM, CCR4, EFNB1, FOXO3, KDR, SLCA4 | |
Females | Hematologic development and function | P values: <0.01 to <0.0001, N=12 genes: BCL2, CD28, CMKLR1, COMMD3-BMI1, FST, MAPK10, PDCD1, PLTP, PRKCH, RAD52, TMEM102, TNFSF4 |
Immune cell trafficking | P values: <0.01 to <0.0001, N=8 genes: BCL2, CD28, CMKLR1, MAPK10, PDCD1, PLTP, TMEM102, TNFSF4, | |
Lymphoid tissue structure and development | P values: <0.01 to <0.0001, N=7 genes: BCL2, CD28, COMMD3-BMI1, FST, PDCD1, PRKCH, TNFSF4 | |
Digestive system development and function | P values: <0.01 to <0.0002, N=7 genes: BCL2, COMMD3-BMI1, FST, GSKIP, NECTIN3, PDCD1, PTK6 | |
Humoral immune response | P values: <0.01 to <0.0002, N=4 genes: BCL2, CD28, PDCD1, TNFSF4 | |
Males | Nervous system development and function | P values: <0.04 to <0.0008, N=6 genes: BRINP1, FOXO3, GPM6B, SLC39A13, TNFRSF21, XPC |
Cardiovascular system development and function | P values: <0.05 to <0.003, N=7 genes: ACKR2, ARHGEF12, BLM, CDH13, FOXO3, MAP1S, MYL9 | |
Connective tissue development and function | P values: <0.05 to <0.003, N=8 genes: ARHGEF12, BLM, BRINP1, CDH13, FOXO3, NACA, SLC39A13, TGIF1 | |
Embryonic development | P values: <0.05 to <0.003, N=6 genes: BLM, KLF3, NDUFAB1, SLC39A13, TGIF1, ZNF613 | |
Hair and skin development and function | P values: <0.05 to <0.003, N=4 genes: ARHGEF12, CDH13, FOXO3, XPC |
For the female cohort, the most statistically significant differentially expressed developmental networks included hematologic development and function, immune cell trafficking, lymphoid tissue structure and development, digestive system development, and humoral immune response. Genes within these pathways are associated with atypical neurogenesis of the intestine, tooth development (i.e., root development, incisor development), development of the secondary and hard palate and an increase of the intestinal villus (31,32).
For the male cohort, the most statistically significant differentially expressed developmental networks included nervous system development and function, cardiovascular system development and function, connective tissue development and function, embryonic development, and hair and skin development and function. Differentially-expressed genes within these networks have been associated with abnormal myelin sheath development, decreased size of the olfactory bulb, dentate gyrus, and the anterior commissure, as well as abnormal morphology of CA1 pyramidal neurons in the hippocampus, a key component in memory (33-35).
Discussion
To date, next-generation sequencing (NGS) platforms have largely been utilized in the neonatal population for either whole genome or whole exome sequencing in critically ill newborns or those with suspected monogenetic disorders (36-40). However, the vast majority of infants born prematurely are neither affected by genetic mutations nor syndromes. Rather, neonatal morbidities are largely a result of disrupted developmental pathways that are a direct consequence of preterm birth. Thus, applying NGS technology to explore real-time gene expression in these at-risk infants holds great potential for furthering our understanding of the molecular mechanisms of neonatal disease and personalizing treatment strategies geared to the individual. To our knowledge, this is the first study to perform high-throughput RNASeq on the premature newborn to better understand the biological mechanisms associated with oral feeding success. We have demonstrated that this approach provides a near real-time window into ongoing development, identifies sex-specific pathways and biological networks associated with impaired oral feeding, and provides caregivers with important opportunities to personalize care and target treatment strategies based upon an infant’s sex and individual gene expression profile.
While infants in the study did have varying gestational ages, ultimately all will need to achieve oral feeding success prior to discharge home. Understanding specific pathways involved in that maturation, irrespective of gestational or post menstrual ages, will be essential in order to develop personalized care approaches to improve feeding outcomes and potentially reduce length of stay in the hospital. When performing a combined comparative systems biology analysis between all successful and unsuccessful oral feeders, developmental pathways involving the nervous, tissue and embryonic systems appear to play a key role in oral feeding. Specifically, pathways involved in cranial nerve (CN) development (CN I, III and IV), sensory integration, and facial development were all identified as being differentially expressed between successful and unsuccessful oral feeders. These pathways are not only biologically relevant but have been shown previously by our group to be essential for oral feeding (11,41). In our prior work, we demonstrated that expression profiles of genes involved in olfactory (PLXNA1) and vision (NPHP4), as well as facial development (WNT3) (11), and cranial nerves (41), predict oral feeding maturation in the newborn. The prospective validation of the importance of these developmental pathways further substantiates their critical role in oral feeding. However, when separated by sex, males and females of similar GAs, PMAs and weight revealed distinct salivary profiles, suggesting that neonates may follow a sex-specific, developmental time course towards oral feeding success.
Clinically, it is well established that female infants will achieve oral feeding competency prior to males of the same GAs and PMAs (12). In fact, sex-specific maturation of oral motor function and development has been seen as early as 15 weeks’ gestation (42). However, the molecular mechanisms responsible for these findings are poorly understood. In the current study, male infants who could not successfully feed were more affected than their female counterparts by nervous system aberrations, particularly as it related to memory and learning. Conversely, unsuccessful female oral feeders were more affected by structural impairments involving intestinal, tooth and palate development compared to males of similar birth weights, GAs and PMAs.
Comparative analyses between male successful and unsuccessful oral feeders highlighted differential expression of genes involved in the neurogenesis of the hippocampus, the migration and morphology of the Cajal-Reelin neurons (43,44), and the morphology of the hippocampal CA1 regions. Interestingly, reelin secreting neurons are located in the marginal zone of the neocortex and the hippocampus and have been an area of investigation in the setting of memory disorders, including Alzheimer’s disease (45). In addition, CA1 regions of the hippocampus are required for contextual memory retrieval, detailed episodic memories, and normal myelination (46). Thus, these data suggest that memory and learning may be delayed or impaired in some male infants struggling to orally feed. Conversely, unsuccessful female oral feeders had gene expression profiles associated with abnormal hard and secondary palate development, as well as disrupted morphology and neurogenesis of the intestine. None of these infants displayed palate malformations. Rather, these data suggest a delay of infant palate maturation, believed to be essential for proper oral feeding, may be a limiting factor to oral feeding success (11). Thus, by applying the RNASeq platform to noninvasively obtained saliva samples, we are able to see clear sex-specific developmental aberrations, allowing us to move beyond merely reporting epidemiological associations and clinical findings, and delve much deeper into the biological mechanisms that are potentially responsible for them.
Clinical applications
Currently, there are several clinical tools available to improve feeding outcomes in the newborn (47-49). However, each device or intervention targets specific developmental pathways, albeit sensory integration or oral motor development. As such, these tools can only best be utilized when applied in a directed fashion to address developmental delays specific to the newborn. Applying high-throughput sequencing technology to explore gene expression allows us to circumvent this limitation. For example, in order to expedite oral feeding and/or treat developmental delays, increased kangaroo care with parents could be utilized in infants lagging in sensory integration (49), while the use of the FDA-approved NTrainer System®, designed to improve nutritive sucking, could be used in infants with delayed facial or neurodevelopment (47). Understanding these differences is essential to developing and implementing targeted and personalized therapies to improve feeding and associated long-term outcomes. Blindly providing treatment strategies to infants struggling to orally feed without a clear understanding of their developmental status, diminishes the effectiveness of the therapy. Rather, in order to truly inform care and improve outcomes, we must be able to successfully integrate near real-time monitoring of neonatal development into newborn care in order to provide targeted, sex-specific, and individualized therapies. While the feasibility of integrating RNASeq analysis of neonatal salivary transcripts into neonatal care may be limited by cost, time and the required expertise in downstream bioinformatic analyses, data generated by this approach may inform the design of a user friendly, cost-effective, targeted array or multiplex RT-qPCR platform for rapid assessment of the developmental feeding stage of the newborn.
Limitations
One of the most appealing aspects of this research is that the data were derived from approximately 10 µL to 20 µL of noninvasively collected whole saliva. However, given the low volume and starting total RNA concentrations in each sample, we could not perform confirmation testing of the RNASeq data with RT-qPCR. Emerging data suggests that this additional step may not be required (50), however, prospective confirmatory studies should be performed in larger cohorts to assess applicability of findings.
In addition, there are unique issues to consider when utilizing whole saliva on the RNASeq platform, including the impact of cellular material on sequence reads, as well as the effect of microbial debris (51). In the current study, there is a wide range of alignment rates between samples, most likely due to varying microbial genomic contamination that does not map to the human genome and or degraded RNA. Nevertheless, this pilot study serves as a proof of principle that neonatal saliva samples are amenable to the RNASeq platform for hypothesis discovery. In order to improve performance on the RNASeq plaform, our laboratory recently published on techniques to optimize output and alignment rates of neonatal saliva on the RNASeq platform, including the use of specific library preparation kits to minimize interference of microbes on the platform (52). Accounting for varying rates of alignment to the human genome by considering the degraded quality of RNA found in saliva, varying cellular contents, small sample volumes, and the bacterial sequences that comprise the oral microbiome will be essential to ensure the integrity of future studies.
Every attempt was made to match successful and unsuccessful oral feeders based upon their sex, gestational and post-menstrual ages. Although none of the demographic data achieved statistical significance, it is possible that differences in both gestational and postmenstrual ages may have skewed the data. In addition, female infants were also shown to have dysregulation of pathways involved in hematopoiesis and immune response. While it is possible that genes within these pathways play a role in oral feeding or gut development, it should be noted that the most common cell types in human saliva are epithelial cells, leukocytes and erythrocytes (53). While future research will need to be conducted to determine what roles, if any, these pathways may play in oral feeding maturity, it is possible that differential cell counts within whole saliva may have resulted in these findings. Finally, our limited sample size makes it unlikely that these data can be universally applied to all newborns across varying PMAs. Nevertheless, our findings continue to contribute to an expanding body of literature demonstrating the biological complexity, as well as sex-specific, time sensitive and distinct maturation, of developmental pathways involved in oral feeding competency in the neonate.
Conclusions
RNASeq of gene transcripts present in neonatal saliva to assess oral feeding competency is feasible, informative and provides near real-time information regarding ongoing development in the neonate. Results of this study reveal that unsuccessful male feeders have delayed nervous system and memory development, while unsuccessful female oral feeders of similar GAs, PMAs and birth weights were more affected by delayed facial structural and gastrointestinal development. While males and females are known to have distinct timelines for the development of oral feeding maturation, integrating gene expression sequencing platforms into neonatal care will allow us to better understand these differences at an organ system level. This approach to noninvasive assessment of the newborn may be used to develop targeted and personalized treatment strategies for the millions of infants affected by oral feeding difficulties born each year. Importantly, this proof of principle study lays the foundation for the assessment of a multitude of other morbidities affecting the preterm newborn and holds significant promise for improving care and outcomes for this vulnerable population.
Acknowledgments
Funding: Tufts Medical Center Institutional Support (Dr. Jill Maron); NICHD R01 HD086088 (PIs: Dr. Steven Barlow and Dr. Jill Maron).
Footnote
Provenance and Peer Review: This article was commissioned by the Guest Editor (Steven M. Barlow) for the series “Neonatal Feeding and Developmental Issues” published in Pediatric Medicine. The article has undergone external peer review.
Reporting Checklist: The authors have completed the STROBE reporting checklist. Available at https://pm.amegroups.com/article/view/10.21037/pm-21-45/rc
Data Sharing Statement: Available at https://pm.amegroups.com/article/view/10.21037/pm-21-45/dss
Conflicts of Interest: All authors have completed the ICMJE uniform disclosure form (available at https://pm.amegroups.com/article/view/10.21037/pm-21-45/coif). The series “Neonatal Feeding and Developmental Issues” was commissioned by the editorial office without any funding or sponsorship. JLM reports that she has received funding for research through Tufts Medical Center and NICHD helped to fund this study, but both Tufts Medical Center and NICHD had no role in the design and conduct of the study; collection management analysis and interpretation of the data; preparation, review or approval of the manuscript; and decision to submit the manuscript for publication. The authors have no other conflicts of interest to declare.
Ethical Statement: The authors are accountable for all aspects of the work in ensuring that questions related to the accuracy or integrity of any part of the work are appropriately investigated and resolved. The study was conducted in accordance with the Declaration of Helsinki (as revised in 2013). This prospective, observational, single-center study was conducted from 2014 to 2017 in the Tufts Medical Center NICU with approval by the Tufts Medical Center Institutional Review Board. The two review panels registered under this IORG are associated with the Tufts Health Sciences Human. Research Protection Program: The IRB # for IRB-RED is IRB00000577, the IRB # for IRB-BLUE is IRB00001236 and the IRB study protocol is #8349. Informed consent was taken from all parents of participating infants.
Open Access Statement: This is an Open Access article distributed in accordance with the Creative Commons Attribution-NonCommercial-NoDerivs 4.0 International License (CC BY-NC-ND 4.0), which permits the non-commercial replication and distribution of the article with the strict proviso that no changes or edits are made and the original work is properly cited (including links to both the formal publication through the relevant DOI and the license). See: https://creativecommons.org/licenses/by-nc-nd/4.0/.
References
- Hawdon JM, Beauregard N, Slattery J, et al. Identification of neonates at risk of developing feeding problems in infancy. Dev Med Child Neurol 2000;42:235-9. [Crossref] [PubMed]
- Mizuno K, Ueda A. The maturation and coordination of sucking, swallowing, and respiration in preterm infants. J Pediatr 2003;142:36-40. [Crossref] [PubMed]
- Lau C. Development of oral feeding skills in the preterm infant. Arch Pediatr 2007;14:S35-41. [Crossref] [PubMed]
- Mizuno K, Ueda A. Neonatal feeding performance as a predictor of neurodevelopmental outcome at 18 months. Dev Med Child Neurol 2005;47:299-304. [Crossref] [PubMed]
- Jadcherla SR, Khot T, Moore R, et al. Feeding Methods at Discharge Predict Long-Term Feeding and Neurodevelopmental Outcomes in Preterm Infants Referred for Gastrostomy Evaluation. J Pediatr 2017;181:125-130.e1. [Crossref] [PubMed]
- Samara M, Johnson S, Lamberts K, et al. Eating problems at age 6 years in a whole population sample of extremely preterm children. Dev Med Child Neurol 2010;52:e16-22. [Crossref] [PubMed]
- da Costa SP, van der Schans CP. The reliability of the Neonatal Oral-Motor Assessment Scale. Acta Paediatr 2008;97:21-6. [Crossref] [PubMed]
- Crowe L, Chang A, Wallace K. Instruments for assessing readiness to commence suck feeds in preterm infants: effects on time to establish full oral feeding and duration of hospitalisation. Cochrane Database Syst Rev 2012;CD005586. [PubMed]
- Crowe L, Chang A, Wallace K. Instruments for assessing readiness to commence suck feeds in preterm infants: effects on time to establish full oral feeding and duration of hospitalisation. Cochrane Database Syst Rev 2016;CD005586. [Crossref] [PubMed]
- Azuma D, Maron J. Individualizing Oral Feeding Assessment and Therapies in the Newborn. Res Rep Neonatol 2020;10:23-30. [Crossref]
- Maron JL, Hwang JS, Pathak S, et al. Computational gene expression modeling identifies salivary biomarker analysis that predict oral feeding readiness in the newborn. J Pediatr 2015;166:282-8.e5. [Crossref] [PubMed]
- Van Nostrand SM, Bennett LN, Coraglio VJ, et al. Factors influencing independent oral feeding in preterm infants. J Neonatal Perinatal Med 2015; [Epub ahead of print]. [Crossref] [PubMed]
- Jackson BN, Kelly BN, McCann CM, et al. Predictors of the time to attain full oral feeding in late preterm infants. Acta Paediatr 2016;105:e1-6. [Crossref] [PubMed]
- Fábryová H, Celec P. On the origin and diagnostic use of salivary RNA. Oral Dis 2014;20:146-52. [Crossref] [PubMed]
- Kaczor-Urbanowicz KE, Martin Carreras-Presas C, Aro K, et al. Saliva diagnostics - Current views and directions. Exp Biol Med (Maywood) 2017;242:459-72. [Crossref] [PubMed]
- Maron JL, Johnson KL, Dietz JA, et al. Neuropeptide Y2 receptor (NPY2R) expression in saliva predicts feeding immaturity in the premature neonate. PLoS One 2012;7:e37870. [Crossref] [PubMed]
- Ludwig SM, Waitzman KA. Changing feeding documentation to reflect infant-driven feeding practice. Newborn Infant Nurs Rev 2007;7:155-60. [Crossref]
- Dietz JA, Johnson KL, Wick HC, et al. Optimal techniques for mRNA extraction from neonatal salivary supernatant. Neonatology 2012;101:55-60. [Crossref] [PubMed]
- Park NJ, Li Y, Yu T, et al. Characterization of RNA in saliva. Clin Chem 2006;52:988-94. [Crossref] [PubMed]
- Schroeder A, Mueller O, Stocker S, et al. The RIN: an RNA integrity number for assigning integrity values to RNA measurements. BMC Mol Biol 2006;7:3. [Crossref] [PubMed]
- O'Reilly E, Baccelli F, De Veciana G, et al. End-to-End Optimization of High-Throughput DNA Sequencing. J Comput Biol 2016;23:789-800. [Crossref] [PubMed]
- Bentley DR, Balasubramanian S, Swerdlow HP, et al. Accurate whole human genome sequencing using reversible terminator chemistry. Nature 2008;456:53-9. [Crossref] [PubMed]
- Deorowicz S, Grabowski S. Compression of DNA sequence reads in FASTQ format. Bioinformatics 2011;27:860-2. [Crossref] [PubMed]
- Trapnell C, Roberts A, Goff L, et al. Differential gene and transcript expression analysis of RNA-seq experiments with TopHat and Cufflinks. Nat Protoc 2012;7:562-78. [Crossref] [PubMed]
- Ghosh S, Chan CK. Analysis of RNA-Seq Data Using TopHat and Cufflinks. Methods Mol Biol 2016;1374:339-61. [Crossref] [PubMed]
- Krämer A, Green J, Pollard J Jr, et al. Causal analysis approaches in Ingenuity Pathway Analysis. Bioinformatics 2014;30:523-30. [Crossref] [PubMed]
- Nguyen TM, Shafi A, Nguyen T, et al. Identifying significantly impacted pathways: a comprehensive review and assessment. Genome Biol 2019;20:203. [Crossref] [PubMed]
- Dunn W Jr, Burgun A, Krebs MO, et al. Exploring and visualizing multidimensional data in translational research platforms. Brief Bioinform 2017;18:1044-56. [PubMed]
- Deák VA, Skroblin P, Dittmayer C, et al. The A-kinase Anchoring Protein GSKIP Regulates GSK3β Activity and Controls Palatal Shelf Fusion in Mice. J Biol Chem 2016;291:681-90. [Crossref] [PubMed]
- Haegebarth A, Bie W, Yang R, et al. Protein tyrosine kinase 6 negatively regulates growth and promotes enterocyte differentiation in the small intestine. Mol Cell Biol 2006;26:4949-57. [Crossref] [PubMed]
- Yoshida T, Miyoshi J, Takai Y, et al. Cooperation of nectin-1 and nectin-3 is required for normal ameloblast function and crown shape development in mouse teeth. Dev Dyn 2010;239:2558-69. [Crossref] [PubMed]
- Bush JO, Soriano P. Ephrin-B1 forward signaling regulates craniofacial morphogenesis by controlling cell proliferation across Eph-ephrin boundaries. Genes Dev 2010;24:2068-80. [Crossref] [PubMed]
- Halfter W, Dong S, Yip YP, et al. A critical function of the pial basement membrane in cortical histogenesis. J Neurosci 2002;22:6029-40. [Crossref] [PubMed]
- Werner HB, Krämer-Albers EM, Strenzke N, et al. A critical role for the cholesterol-associated proteolipids PLP and M6B in myelination of the central nervous system. Glia 2013;61:567-86. [Crossref] [PubMed]
- Schmidt-Strassburger U, Schips TG, Maier HJ, et al. Expression of constitutively active FoxO3 in murine forebrain leads to a loss of neural progenitors. FASEB J 2012;26:4990-5001. [Crossref] [PubMed]
- Meng L, Pammi M, Saronwala A, et al. Use of Exome Sequencing for Infants in Intensive Care Units: Ascertainment of Severe Single-Gene Disorders and Effect on Medical Management. JAMA Pediatr 2017;171:e173438. [Crossref] [PubMed]
- Miller NA, Farrow EG, Gibson M, et al. A 26-hour system of highly sensitive whole genome sequencing for emergency management of genetic diseases. Genome Med 2015;7:100. [Crossref] [PubMed]
- Petrikin JE, Willig LK, Smith LD, et al. Rapid whole genome sequencing and precision neonatology. Semin Perinatol 2015;39:623-31. [Crossref] [PubMed]
- Maron JL, Kingsmore SF, Wigby K, et al. Novel Variant Findings and Challenges Associated With the Clinical Integration of Genomic Testing: An Interim Report of the Genomic Medicine for Ill Neonates and Infants (GEMINI) Study. JAMA Pediatr 2021;175:e205906. [Crossref] [PubMed]
- Kallankari H, Huusko JM, Kaukola T, et al. Cerebral Palsy and Polymorphism of the Chemokine CCL18 in Very Preterm Children. Neonatology 2015;108:124-9. [Crossref] [PubMed]
- Maron JL, Johnson KL, Rocke DM, et al. Neonatal salivary analysis reveals global developmental gene expression changes in the premature infant. Clin Chem 2010;56:409-16. [Crossref] [PubMed]
- Miller JL, Macedonia C, Sonies BC. Sex differences in prenatal oral-motor function and development. Dev Med Child Neurol 2006;48:465-70. [Crossref] [PubMed]
- Ji J, Maren S. Differential roles for hippocampal areas CA1 and CA3 in the contextual encoding and retrieval of extinguished fear. Learn Mem 2008;15:244-51. [Crossref] [PubMed]
- Booler HS, Williams JL, Hopkinson M, et al. Degree of Cajal-Retzius Cell Mislocalization Correlates with the Severity of Structural Brain Defects in Mouse Models of Dystroglycanopathy. Brain Pathol 2016;26:465-78. [Crossref] [PubMed]
- Joo Y, Schumacher B, Landrieu I, et al. Involvement of 14-3-3 in tubulin instability and impaired axon development is mediated by Tau. FASEB J 2015;29:4133-44. [Crossref] [PubMed]
- Bartsch T, Döhring J, Rohr A, et al. CA1 neurons in the human hippocampus are critical for autobiographical memory, mental time travel, and autonoetic consciousness. Proc Natl Acad Sci U S A 2011;108:17562-7. [Crossref] [PubMed]
- Poore M, Zimmerman E, Barlow SM, et al. Patterned orocutaneous therapy improves sucking and oral feeding in preterm infants. Acta Paediatr 2008;97:920-7. [Crossref] [PubMed]
- Chorna OD, Slaughter JC, Wang L, et al. A pacifier-activated music player with mother's voice improves oral feeding in preterm infants. Pediatrics 2014;133:462-8. [Crossref] [PubMed]
- Giannì ML, Sannino P, Bezze E, et al. Does parental involvement affect the development of feeding skills in preterm infants? A prospective study. Early Hum Dev 2016;103:123-8. [Crossref] [PubMed]
- Coenye T. Do results obtained with RNA-sequencing require independent verification? Biofilm 2021;3:100043. [Crossref] [PubMed]
- Conesa A, Madrigal P, Tarazona S, et al. A survey of best practices for RNA-seq data analysis. Genome Biol 2016;17:13. [Crossref] [PubMed]
- Yen E, Kaneko-Tarui T, Maron JL. Technical Considerations and Protocol Optimization for Neonatal Salivary Biomarker Discovery and Analysis. Front Pediatr 2020;8:618553. [Crossref] [PubMed]
- Cianga C, Antohe I, Zlei M, et al. Saliva leukocytes rather than saliva epithelial cells represent the main source of DNA. Revist Romana de Medicine de Laborator 2016;24:31-44. [Crossref]
Cite this article as: Khanna P, Jenney K, Tai AK, Maron JL. Salivary RNA sequencing highlights a sex-specific developmental time course towards oral feeding maturation in the newborn. Pediatr Med 2022;5:11.